The neural networks we have developed simplify structure determination and improve learning ability by applying stochastic/statistical theory to determine the structure of the networks. Uniquely constructed neural networks include the Log-linearized Gaussian Mixture Network (LLGMN) and the Recurrent-LLGMN (R-LLGMN). LLGMN corresponds to a mixed normal distribution, which is a statistical model, and R-LLGMN corresponds to the introduction of a Hidden Markov Model (HMM) that effectively models time series signals. The Reduced-Dimension-LLGMN (RD-LLGMN) extracts and classifies the principal components from multivariate data, whereas the Hierarchical-LLGMN (H-LLGMN) constructs a hierarchical system of LLGMNs for efficient modeling. LLGMN is suitable for modeling steady-state patterns with little time variation, and R-LLGMN is suitable for modeling nonstationary patterns that change dynamically over time.
Resarch topics
Novel Probabilistic Neural Network
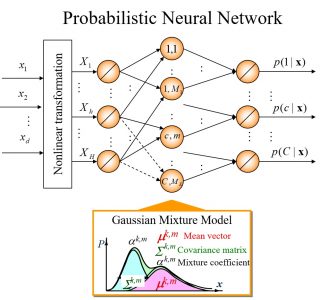
References
1. A Log-Linearized Gaussian Mixture Network and Its Application to EEG Pattern Classification, T.Tsuji, O.Fukuda, H.Ichinobe and M.Kaneko, IEEE Transactions on Systems, Man, and Cybernetics-Part C: Applications and Reviews, Vol. 29, No. 1, pp.60-72, February, 1999.
2. A Recurrent Log-linearized Gaussian Mixture Network, Toshio Tsuji, Nan Bu, Makoto Kaneko, Osamu Fukuda
IEEE Transactions on Neural Networks, Vol.14, No.2, pp.304-316, March 2003.